Pre-Conference Workshops
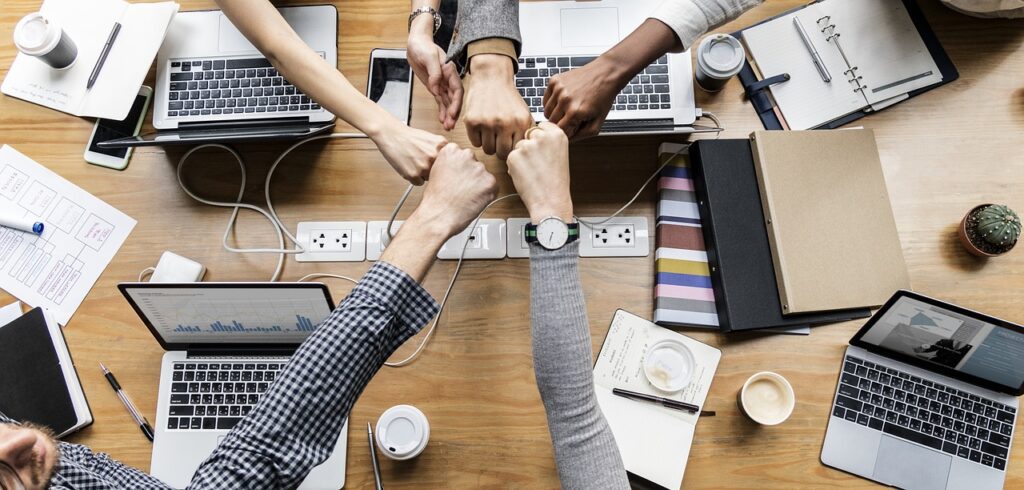
We offer several pre-conference workshops, on the weekend before the conference. A wide range of themes and skills will be covered, do not miss it!
Saturday 13 and Sunday 14 July 2024
1. NIMBLE for ecology: MCMC, particle filtering, Hamiltonian Monte Carlo, and Laplace approximation
Date: Saturday 13
Length: Half day
Instructors: Daniel Turek (Williams College, Williamstown, USA), Wei Zhang (University of Glasgow, UK), Perry De Valpine (University of California, Berkeley, USA)
Overview: Those who want to learn a general and extensible platform for hierarchical statistical modelling, including custom distributions for ecological models and the new features of HMC and Laplace approximation. Attendees should be familiar with writing models in the BUGS language (JAGS, BUGS or NIMBLE).
2. Fitting Integrated Distribution Models with PointedSDMs
Date: Saturday 13
Length: half day
Instructors: Bob O’Hara, Philip Mostert, Ron Tugonov, Kwaku Adjei (Norwegian University of Science and Technology, Trondheim, Norway)
Overview : The workshop will introduce integrated distribution modelling and the PointedSDMs software. This will include how to format the data, and fit and adapt models with different observation processes. The workshop will be interactive, so attendees will be able to practice fitting the models and get help working with their own data.
3. Using GAMs for analyzing movement data
Date: Sunday 14
Length: Full day
Instructors: Eric Pedersen (Concordia University, Montreal, Canada) and Fonya Irvine (Concordia University, Montreal, Canada). Natasha Klappstein (Dalhousie University, Halifax, Canada) and Théo Michelot (Dalhousie University, Halifax, Canada).
Overview: This one-day workshop will focus on the application of generalised additive models (GAMs) to animal movement data, including theoretical background, implementation, and interpretation. Participants are expected to be familiar with simpler regression models and with the statistical software R.
The morning will present a general overview of GAMs (with a focus on animal movement applications), including:
- basis functions, penalisation
- types of smoothers and random effects
- introduction to mgcv
- smooth visualisation/interpretation
The afternoon will show how to use GAMs to incorporate non-linear and random effects in two widely-used animal movement models:
- step selection functions in mgcv
- hidden Markov models in hmmTMB
4. Hidden Marrkov models for movement and accelerometer data
Date: Sunday 14
Length: full day
Organisers: Fanny Dupont (University of British Columbia, Canada), Marco Gallegos Herrada (University of Toronto, Canada), Marie Auger-Méthé (University of British Columbia, Canada), Vianey Leos Barajas (University of Toronto, Canada).
Instructors: Fanny Dupont (University of British Columbia), Marco Gallegos Herrada (University of Toronto), Marie Auger-Méthé, Vianey Leos Barajas
Overview: The workshop aims to demonstrate how hidden Markov models (HMMs) can be used to classify behaviours and identify behaviour-specific habitat associations using a range of movement and biologging data
5. runMCMCbtadjust : an R package facilitating the use of Bayesian Markov Chain(s) Monte-Carlo (MCMCs)
Date: Sunday 14
Length: half day
Instructor: Frédéric Gosselin (UR EFNO, INRAE, Domaine des Barres, France)
Overview : Presentation of a new R package called runMCMCbtadjust (https://cran.r-project.org/web/packages/runMCMCbtadjust/) that can help R users of MCMC models having a more efficient, quality oriented use of these types of models while saving analyst’s and potentially computer time.
6. Joint species distribution modelling with the R-package Hmsc
Date: Sunday 14
Length: full day
Instructors: Otso Ovaskainen (University of Jyväskylä, Finland) and Gleb Tikhonov (University of Helsinki, Finland)
Overview: In this workshop, the participants learn how to apply the Joint Species Distribution Modelling Framework of Hierarchical Modelling of Species Communities (HMSC) with the R-package Hmsc-R. HMSC can be used to model multispecies data on species occurrences/abundances as a function of environmental, spatial and temporal predictors, species traits and taxonomies/phylogenies. The workshop includes brief lectures introducing the conceptual and statistical background of HMSC. The main part of the workshop consists of computer demonstrations showing how to apply Hmsc-R to various types of data, such as hierarchical, spatial and temporal sampling designs, as well as dataset including traits and taxonomical/phylogenetic information. Compared to earlier HMSC courses organized in 2020 and 2022, this course includes as new elements instructions on how to fit HMSC up to 1000 times faster with the newly released high-performance computing module Hmsc-HPC, as well as new methods on how to link HMSC outputs to niche theory by summarizing them into shared and idiosyncratic responses of the species to measured and latent predictors. Participants working already with HMSC on their own data are provided one-to-one support.
Intended audience: PhD students & researchers working with community data (=multispecies data) or developing statistical methods in this area. The course should be interesting for a broad set of ecologist and statistical ecologists.
7. RTMB: Automatic differentiation in R
Date: Sunday 14
Length: Full day
Instructors: Anders Nielsen (DTU – Danish Technical University, Denmark); Ben Bolker (University of McMaster, Canada)
Overview: This workshop will introduce participants to RTMB. RTMB is the latest iteration of TMB (Template Model Builder), a widely used tool for flexible statistical modeling. RTMB’s novel feature is that models are specified in plain R code (rather than C++ code or another special modelling language). Once the model is specified it evaluates function values and derivatives as efficiently as if the model were written in C++. Calculations in RTMB are supported by automatic differentiation, sparse matrices, delta-method calculations, and efficient Laplace approximation, which makes RTMB ideal for maximum likelihood inference in small and large models with and without random effects. Models specified in RTMB can also sampled with MCMC by linking them to Stan. In the course examples and exercises will span non-linear regression, generalized linear models, random effects, state-space, and spatio-temporal models with flexible spatial mesh structure (as known from INLA).